2024年12月14日 10:00 天津
人工智能训练与负责任的人工智能
避免LLM生成有偏见、有害的内容
AI Training and Responsible AI
Preventing biased and harmful output from LLM
As more and more businesses start using their own Large Language Models (LLMs), responsible AI becomes a critical concern. A key part of responsible AI is using AI training and data validation to prevent any hateful, intolerant, or biased AI content generation. This kind of content can be harmful and contribute to overall social problems, including (but not limited to):
-
Spreading hate speech
-
Marginalizing certain groups or communities
-
Causing emotional distress
随着越来越多的企业开始使用自己的大型语言模型 (LLM),如何负责任地应用人工智能逐渐成为了一项重要的议题。实现这一目标的关键在于利用人工智能训练和数据检验来避免其生成充满仇恨、有歧视性或带有偏见的内容。这样的内容害处极大,还有可能引发大范围社会问题,包括(但不限于):
-
传播仇恨言论
-
边缘化特定群体
-
造成精神压力
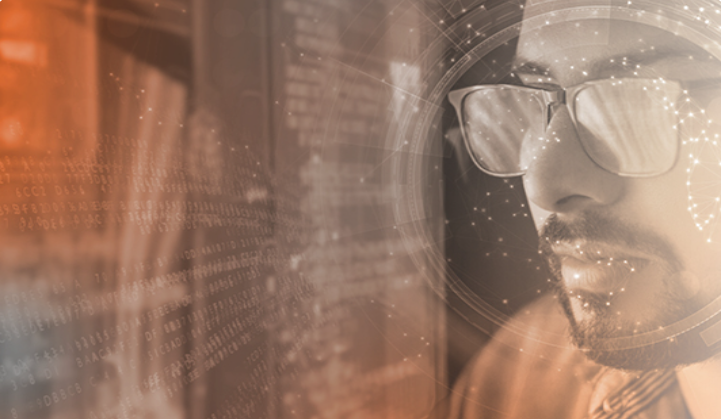
五大策略:确保负责任地使用人工智能并避免生成有害内容
Consider implementing all, or at least some, of these tactics to ensure your AI output isn’t unintentionally biased, racist, misogynistic, or simply offensive or culturally taboo. For optimal results, work with a diverse group of people throughout the AI training and monitoring process. They will bring a wider and stronger base of knowledge. Consider working with AI training experts, who combine expertise in AI, sociocultural norms, industries, and linguistics. Lastly, some companies may set policies for AI developers and users. These policies articulate consequences for misuse of an AI system. They motivate everyone to help ensure an AI never creates harmful or offensive content.
请考虑全部采纳,或至少部分采纳这些策略,以确保人工智能不会无意中生成带有偏见、种族歧视、女性歧视、冒犯他人或触犯文化禁忌的内容。为了取得最佳成果,需要让不同背景的人群参与到人工智能的训练和监督中,以获得更为广泛和坚实的知识基础。可以考虑与人工智能培训专家合作,他们集合了人工智能、社会文化规范、行业和语言学方面的专长。最后,一些公司可能会为人工智能开发者和用户制定政策。这些政策阐明了滥用人工智能系统的后果,鼓励每一个人都出力保证人工智能永远不会创造有害或冒犯性的内容。
策略 1:数据处理
When conducting AI training, proper data collection is crucial. Companies should take a two-pronged approach. Firstly, filter out data from sources that may include problematic viewpoints. The second step is ensuring the training data for an LLM represents a diverse array of voices and perspectives. If the content is multilingual or comes from differing locations or cultures, it may help to have local or linguistic experts assist with these tasks.
在进行人工智能培训时,收集恰当的数据至关重要。为此,公司应采取双管齐下的方法。首先,从来源中筛除可能包含错误观点的数据。其次,确保大型语言模型的训练数据中包含丰富多样的声音和观点。如果内容包含多种语言或涉及不同地域、文化,邀请当地专家或语言学家协助处理任务可能会有所帮助。
策略 2:制定道德准则
When training AI for ethical output, building an ethical framework is essential. Much like creating a style guide or translation glossary, a company should develop a series of rules and guidelines that it would want all its content to abide by. Use industry standards to help develop the framework, ensuring compliance and better results. These frameworks may need to be expanded and varied for multilingual or cultural work to include new language and social norms or taboos. Companies should also set up protocols and structures for continuous ethical deployment of the AI model.
如果希望人工智能经过训练生成的内容符合道德规范,那么就有必要制定一套道德准则。像编写风格指南或翻译词汇表一样,公司应当制定一系列规则和方针,以确保所有内容都能够遵循这些规范。利用行业标准帮助制定这套准则,确保符合法规的同时也可取得更好的成果。在开展多语言或多文化工作时,这套准则可能需要进一步扩充修改,以适应新的语言、社会规范和禁忌。公司还应制定协议和框架,以道德地持续应用人工智能模型。
Tactic 3: Ethical and Bias Pre-Training
策略 3:道德和偏见预培训
During the pre-training and fine-tuning phases, companies should prioritize bias mitigation techniques. Using the ethical framework mentioned above, the LLM should be taught to identify and avoid creating and consuming biased or offensive content. When testing The LLM during pre-training, it’s essential to use data validation to update data sets with a foundational understanding of ethics and biases. The ethical framework is helpful for this step, as well.
在预训练和微调阶段,公司应当将重点放在减少偏见的技术上。利用上述道德准则,经过训练的大型语言模型应当能够识别并避免生成或接收带有偏见或冒犯性的内容。在预训练阶段测试大型语言模型时,必须使用数据验证来更新数据集,确保其对道德规范和偏见有基本的了解。道德准则在这一步也发挥了很大作用。
During training, consider creating mechanisms that showcase the AI model’s decision-making when identifying and rejecting offensive content. This transparency will help later if there are issues.
在训练中,要考虑建立一套机制,展示人工智能模型在识别和拒绝冒犯性内容时的决策过程。日后一旦出现问题,其透明性将发挥重要作用。
Tactic 4: Continually Monitor Output
策略 4:持续监控生成内容
After training their AI, a company must still continue reviewing output. For mission-critical content, a human reviewer is worth considering to ensure quality and compliance with the ethical framework. This is particularly helpful for content designed for customers who speak different languages and come from other cultures. Moreover, companies may also consider creating opportunities for customers to report offensive content, and incorporate this feedback into continuous fine-tuning efforts.
在对人工智能进行培训后,公司必须持续审查其生成的内容。对于关键内容应考虑引入人工审查,确保这些内容质量过关且符合道德准则。如果内容面向的是多语言、多文化背景的客户,这一点尤为重要。此外,公司还可以考虑为客户提供一条冒犯性内容报告渠道,并根据这些反馈不断改进。
Tactic 5: Retrain as Needed
策略 5:根据需要重复训练
Companies should build retraining into their protocols for a couple of reasons. Firstly, the AI model may not thoroughly “learn” how to correctly apply the ethical framework initially. It may erroneously create offensive content, or the ethical framework itself might be lacking. A second reason for continued retraining is because cultural norms change constantly. While content isn’t offensive today, it could be tomorrow — especially if it’s developed for customers who speak multiple languages or come from other cultures. The more cultures and languages, the more nuances required for an ethical framework.
公司应将重复训练纳入规程,原因有以下几点。首先,人工智能模型最初可能无法完全“掌握”如何准确地应用道德准则,因而错误地生成冒犯性内容,又或者道德准则本身可能尚未完善。其次,文化规范是不断演变的,因此需要不断重复训练。今天看似无害的内容,明天可能就会冒犯到他人——尤其是内容面向说多种语言或来自其他文化的客户时。文化和语种越是丰富,道德准则就越要细致。
原文网址:https://www.lionbridge.com/blog/translation-localization/ai-training-and-responsible-ai/
特别说明:本文内容来自Lionbridge官网
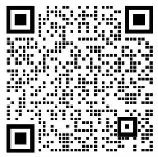
– END –