翻译技术教育与研究 2024年11月28日 07:03 陕西
编者荐语:
本文内容摘译自Slator官网,这项研究不仅实现了在LLM性能评估时的一致性和可比性,还为跨语言NLP的发展注入了新的活力,一起来看看吧~
以下文章来源于国际翻译动态 ,作者钱彦丰
国际翻译动态
研究发现:AI翻译的测试基准
可以很好地测试大语言模型性能
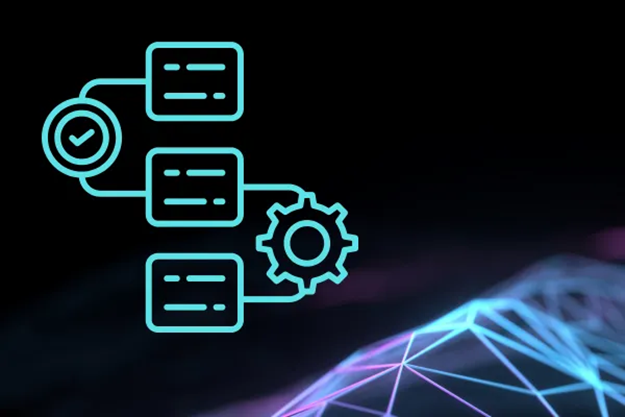
(图片来自Slator官网)
On October 17, 2024, the OpenGPT-X Team released machine-translated versions of five well-known benchmarks in 20 European languages, enabling consistent and comparable evaluation of large language models (LLMs).
2024年10月17日,OpenGPT-X团队对外公布了他们使用机器翻译将5项知名基准翻译成20种欧洲语言,实现了在大型语言模型(LLM)性能评估时的一致性和可比性。
Using these benchmarks, the team evaluated 40 state-of-the-art models across the languages, providing valuable insights into their performance.
通过这些基准测试,该团队评估了20种语言中最先进的40个模型,为其性能测试提供了有价值的信息。
The OpenGPT-X Team highlighted the challenges of evaluating LLM performance consistently across languages. According to the researchers, “evaluating LLM performance in a consistent and meaningful way […] remains challenging, especially due to the scarcity of language-parallel multilingual benchmarks.”
OpenGPT-X团队强调了在跨语言环境下评估大语言模型性能的同时保持一致性所遇到的挑战。根据研究人员的说法,“以一致且有效的方式评估大语言模型性能[…]仍然具有挑战性,尤其是缺乏同语言并行的多语言基准。
They also noted the high costs and time required to create custom benchmarks for each language, which has led to a “fragmented understanding of model performance” across different languages. “Without comprehensive multilingual evaluations, comparisons between languages are often constrained,” they explained, particularly for languages beyond the widely supported English, German, and French.
他们还指出了为每种语言定制相应基准所需的成本和时间都很高,这导致不同语言之间“对模型性能的理解不够完整”。他们解释道:“如果没有全面多语言评估,语言之间的比较往往会受到限制。”特别是那些除英语、德语和法语之外、使用范围不够广范的语言。
To tackle this, the team employed machine-translated versions of widely used datasets, aiming to assess whether such translations could provide scalable and uniform evaluation results.
为了解决这个问题,该团队通过机器来翻译那些广为使用的数据集,利用这些机器翻译的版本,测试其是否可以提供可扩展和统一的性能评估结果。
1
Machine-Translated Benchmarks
as a Reliable Proxy
机器翻译的基准作为可靠代理
Specifically, they translated five well-known datasets — ARC for scientific reasoning, HellaSwag for commonsense reasoning, TruthfulQA for factual accuracy, GSM8K for mathematical reasoning and problem-solving abilities, and MMLU for general knowledge and language understanding — from English into 20 European languages using DeepL.
具体来说,他们使用DeepL将五个著名的数据集——用于科学推理的ARC,用于常识推理的HellaSwag,用于事实准确性的TruthfulQA,用于数学推理和解决问题的能力的GSM8K,用于一般知识和语言理解的MMLU——从英语翻译成20种欧洲语言。
“Our goal is to determine the effectiveness of these translated benchmarks and assess whether they can substitute manually generated ones,” the team stated.
该团队表示,“我们的目标是确定这些翻译基准的有效性,并评估它们是否可以替代人工设置的基准。”
Their findings suggest that machine-translated benchmarks can serve as a “reliable proxy” for human evaluation in various languages.
研究结果表明,机器翻译的基准能够成为在各种语言下人类评估的“可靠代理”。
2
Top Performers and Language
Trends
顶级性能和语言趋势
Using the translated datasets, along with the multilingual FLORES-200 benchmark for translation tasks, the team evaluated 40 models across 21 European languages.
使用翻译后的数据集,以及用于翻译任务的多语言FLORES-200基准,该团队评估了21种欧洲语言的40个模型。
They identified Meta’s Llama-3.1-70B-Instruct and Google’s Gemma-2-27b-Instruct as the top-performing models across multiple tasks. Llama-3.1-70B stood out in knowledge-based tasks, like answering general questions (MMLU) and solving math problems (GSM8K), as well as in commonsense reasoning (HellaSwag) and translation tasks. Meanwhile, Gemma-2-27b-Instruct excelled in scientific reasoning (ARC) and giving factually accurate answers (TruthfulQA).
他们认为Meta的Llama-3.1-70B-Instruct和Google的Gemma-2-27b-Instruct是多个任务中性能最优的模型。Llama-3.1-70B在基于知识的任务中脱颖而出,如回答一般性问题(MMLU),解决数学问题(GSM8K),以及常识推理(HellaSwag)和翻译任务。与此同时,Gemma-2-27b-Instruct在科学推理(ARC)和给出符合事实的答案(TruthfulQA)方面表现出色。
Smaller models like Gemma-2-9b-Instruct, though consistent in common tasks, struggled in specialized domains. The researchers noted, “the capacity of small models might not allow for reliable performance on all languages and specialized knowledge.”
而像Gemma-2-9b-Instruct这样较小的模型,虽然在常规任务中保持一致,但在专业领域中却很难。研究人员指出,“小型模型的容量可能无法在所有语言和专业知识上表现出可靠的性能。
Additionally, high-resource languages like English, German, and French consistently saw better results, while medium-resource languages, such as Polish and Romanian, displayed weaker performance across tasks.
此外,英语、德语和法语等高资源语言的性能一直较好,而波兰语和罗马尼亚语等中资源语言的性能则较弱。
The results are publicly available through the European LLM Leaderboard, a multilingual evaluation platform.
团队将结果公布在一个多语言评估平台——欧洲大语言模型排行榜(European LLM Leaderboard)上。
The team emphasized the broader impact of their work: “By ensuring that LLMs can perform well in languages beyond English or other high-resource languages, we contribute to a more equitable digital landscape.”
该团队强调了这项研究的广泛影响:“通过确保大语言模型能够在除英语等高资源语言之外的语言中表现一样出色,我们为更公平的数字环境做出了贡献。”
To encourage further research, the team has made the machine-translated datasets available to the NLP community. “We aim to foster further research and development in multilingual LLM evaluation, driving improvements in cross-lingual NLP applications,” they concluded.
为了鼓励进一步的研究,该团队将机器翻译的数据集提供给了自然语言处理(NLP)领域研究人员。他们总结道,“我们的目标是促进多语言大语言模型评估的进一步研究和开发,推动跨语言自然语言处理应用程序的改进。”
原文网址:https://slator.com/ai-translated-benchmarks-can-reliably-assess-llm-performance-study-finds/
特别说明:本文内容选自Slator官网,仅供学习交流使用,如有侵权请后台联系小编删除。